Winner of ISEF 2021 uses ZTF public data to discover faint asteroids
A high school student uses machine learning and finds undetected asteroid streaks in ZTF public data
Franklin Wang was one of the thousands high school participants in this year's International Science and Engineering Fair. He was also one of the few winners. His project "The Fast and Inconspicuous: New Near Earth Asteroids Discovered Using Deep Learning and Synthetic Data Are Fainter and Move Faster than Those Previously Discovered" was awarded first prize in the Physics/Astronomy category. Franklin also received the Peggy Scripps Award for Science Communication, as the " finalist who is best able to communicate their project to the lay public, explaining both the science and its potential impact on society".
We chatted with Franklin about his project, his passion for astronomy and his aspirations for the future.
Tell us a bit about yourself, your encounter, and your passion for physics and astronomy
Currently, I am a rising senior at Palo Alto High School and I have had the great fortune of winning 1st place in the Astronomy & Physics Category at Regeneron ISEF. Ever since I was a kid, I’ve always been interested in astronomy. I remember reading about dark matter and dark energy in Scientific American as an elementary school student and being amazed at all the amazing quirks our universe has to offer!
How and why did you choose the project you presented at ISEF?
During middle school, I also gained a strong interest in programming, specifically with machine learning. I made a couple of fun projects, such as a lyrics generator program, but I felt that I could do more with machine learning. That’s when I decided to combine my passion for machine learning and astronomy to work on research into the applications of machine learning to astronomy. Reading the DeepStreaks paper by Dr. Duev got me interested in the specific field of asteroid detection, and that’s where my research really took off.
Can you describe your project in more detail?
In my research, my goal is to use a purely simulated dataset of asteroid streaks to train a machine learning model that learns how to recognize asteroid streaks. These “streaks” correspond to asteroids with a very high rate of apparent motion, causing them to leave trails in telescope exposures. These fast-moving asteroids are of particular interest as they tend to be closer to Earth, and thus may be more dangerous. By using a purely simulated dataset of asteroid streak, I am able to create a larger dataset of streaks than I would be able to with real data, since there are not many available exposures of real asteroid streaks. I also specifically target my dataset towards fainter asteroid streaks, which are harder to find. Through this improved dataset, I have been able to find six streaking asteroids that ZTF missed using just four nights of data.
How did you feel when you heard you won first prize in Physics and a named prize as well?
Right before I heard the prizes being announced, I was actually feeling very sleepy due to the awards being held quite early in the morning. After I heard the awards I was definitely no longer feeling sleepy though. I wasn’t sure what to expect from the awards and so it was a huge surprise when I found out I won first prize. Receiving the Peggy Scripps award for science communication was an even bigger surprise. Overall, I put a lot of time and effort into my project and clearly communicating my research. Seeing all that work pay off was really exciting!
What was the most exciting and most challenging moment during your project?
The most exciting moment definitely was when I found my first undiscovered asteroid streak. Going from zero discoveries to one discovery was extremely exciting, as all the work I put into this research finally started to show results. Although each additional asteroid streak that I found afterward was also very exciting, they were not nearly as exciting as the first one.
For my research, the biggest challenge was trying to reduce the number of false positive detections that my algorithm was returning. In my first few attempts at running my detection pipeline on a full night of data, I was met with tens of thousands of false positives — far too many to go through manually. Figuring out strategies to reduce false positives was a significant challenge, and even now I am still trying to find new ways to reduce false detections.
What’s the most desired professional path you’d like to take in the future?
In the future, I would like to continue pursuing research as a researcher in an academic setting. The many applications of machine learning in astronomy fascinate me and is a topic that I wish to explore further!
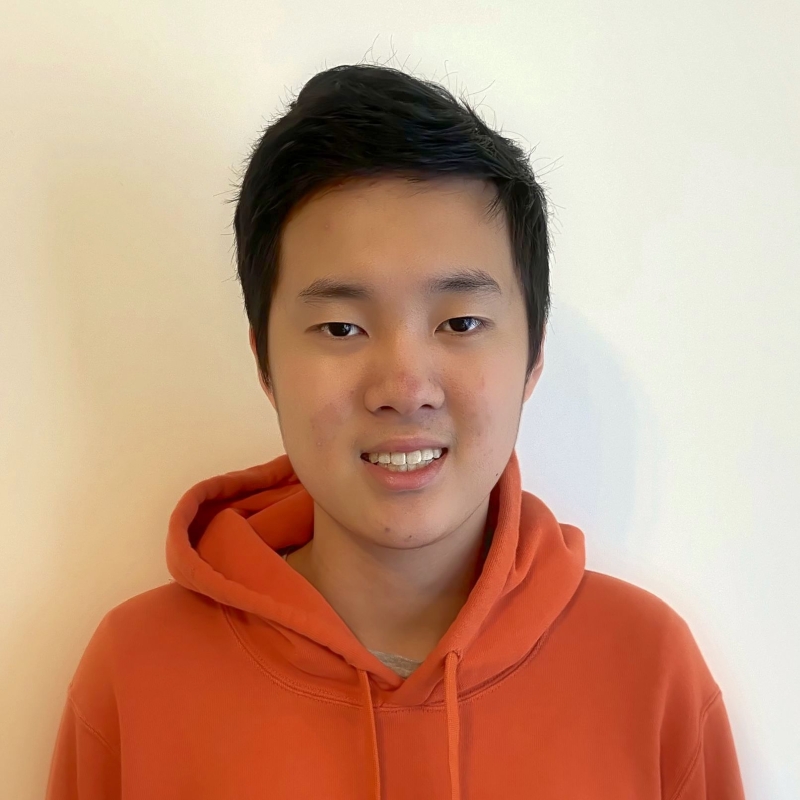
Franklin Wang
Franklin Wang presenting his winning project. Watch time ~5 min.